CI4R Alumni
(Click Names to View Details)
Doctor of Philosophy
Emre Kurtoglu, PhD, The University of Alabama, 2024
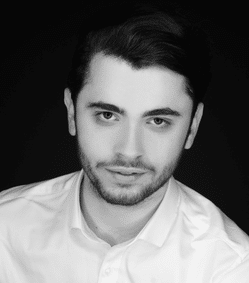
Post-Graduation Affiliation:
Research Scientist
Data Science Platform
Rockefeller University
New York City, NY
Emre Kurtoglu
PhD in Electrical and Computer Engineering, May 2024
Thesis Title: Fully-Adaptive RF Sensing for Non-Intrusive ASL Recognition via Interactive Smart Environments
Abstract: The past decade has seen great advancements in speech recognition for control of interactive devices, personal assistants, and computer interfaces. However, Deaf people and people with hard-of-hearing, whose primary mode of communication is sign language, cannot use voice-controlled interfaces. Although there has been significant work in video-based sign language recognition, video is not effective in the dark and has raised privacy concerns in the Deaf community when used in the context of human ambient intelligence. Radars have recently been started to be used as a new modality that can be effective under the circumstances where video is not. This dissertation conducts a thorough exploration of the challenges in RF-enabled sign language recognition systems. Specifically, it proposes an end-to-end framework to acquire, temporally isolate, and recognize individual signs. A trigger sign detection with an adaptive thresholding method is also proposed. An angular subspace projection method is presented to separate multiple targets at raw data level. An interactive sign language-controlled chess game is designed to enhance the user experience and automate the data collection and annotation process for labor-intensive data collection procedure. Finally, a framework is presented to dynamically adjust radar waveform parameters based on human presence and their activity.
Awards/Honors while @ CI4R:
- Finalist in Best Student Paper Competition, 2024 IEEE Radar Conference, Denver, CO
- Finalist in Best Student Paper Competition, 2022 IEEE Radar Conference, New York City, NY
- UA Graduate Council Fellowship, Aug. 2021 – May2022
- Google Summer of Code, 2021
- Internship at NXP Semiconductors, San Jose, CA, May – Aug. 2023
M. Mahbubur Rahman, PhD, The University of Alabama, 2024
Mohammed Mahbubur Rahman
PhD in Electrical and Computer Engineering, May 2023
Thesis Title: Physics-Aware Deep Learning for Radar-Based Cyber-Physical Human Systems
Abstract (Abridged): Radar is an emerging sensing modality utilized in Cyber-Physical Systems (CPS), where it has applications in health and safety CPS, CPS for gesture/sign language-driven smart environments, and automotive CPS. However, these CPHS scenarios present various sensing challenges, which in turn lead to various deep learning challenges, like training under insufficient support samples, sensors/ aspect angles invariant feature learning for RF sensors interoperability, open-set problems for recognizing diverse human motions without prior training data and decoupling individual motions from a multi-person scenario. Conventional data-driven deep learning approaches require vast amounts of training data for each scenario, but gathering large volumes of RF data is a major challenge in itself. To address these issues, this dissertation proposes physics-aware deep learning solutions that leverage physical prior knowledge and operate effectively with limited measured data. In particular,
1. Physics-aware generative adversarial networks for improved synthesis of human RF signatures as training data, which leverage models of human kinematics, are developed.
2. The question of how to achieve interoperability between multi-frequency RF sensors to mitigate training data deficiency is explored. Two approaches namely, domain adaptation, and cross-modal fusion are proposed to address this problem.
3. A physics-aware RF-based human pose estimation framework that recognizes diverse human motions and facilitates gait analysis and fall risk assessments in elderly care and nursing homes is developed
The efficacy of these solutions is demonstrated through examples of the gross body human activity, such as ambulatory human motion recognition for health and safety CPS applications, and fine-grained human motions, such as American Sign Language (ASL) recognition for smart deaf space design. Notably, this dissertation is the first to conduct a study on RF-based ASL recognition, achieving state-of-the-art classification performance for 100-word fluent ASL recognition. Thus, this dissertation provides innovative and practical solutions to the challenges of radar-enabled CPHS, leveraging physics-aware techniques to enable effective learning with limited data and advance the field toward more accurate and reliable sensing.
Awards/Honors while @ CI4R:
- 3rd Place in Best Student Paper Competition, 2021 IEEE Radar Conference, Atlanta, GA
- UA Graduate Council Fellowship, Aug. 2020 – May 2021
- Internship, Mitsubishi Electric Research Laboratories, Summer 2022
Master of Science
Trevor Macks, M.S., The University of Alabama, 2020
Trevor Macks
M.S. in Electrical and Computer Engineering, May 2020
Thesis Title: American Sign Language Recognition Using Adversarial Learning in a Multi-Frequency RF Sensor Network
Abstract: Human computer interaction (HCI) based technologies, such as Alexa or Siri, have become increasingly prevalent in the daily lives of American citizens. However, access to these technologies is gated by the need to communicate with spoken commands, which subsequently precludes the Deaf community from benefitting from the quality of life improvements they provide. Current approaches to providing HCI technologies or ASL conversion largely rely on video and image processing techniques, haptic gloves, and wi-fi based systems. However, wearables restrict users from engaging in their normal daily activities, while video raises privacy concerns. To help ASL compatible HCI technology, we propose a multi-frequency RF sensing network for the recognition of a basic lexicon of signs. Two datasets were acquired for training deep models: a native signing dataset and an imitation signing dataset. The native dataset is small, and was collected from Deaf individuals. Our imitation dataset is larger, and was collected from hearing individuals prompted by copysigning videos. We show that imitation data cannot be used in lieu of native ASL data for training and benchmarking classifiers because the two datasets possess disparate distributions in feature space. Alternatively, we investigate adversarial learning as a means for mitigating the challenge of insufficient training data. Cross frequency training is one option for augmenting the training dataset which suffers from severe performance degradation when data from one frequency is used to pre-train a network for classification of data at another frequency. We show that data synthesized using Generative Adversarial Networks (GANs) can be used to reduce but not completely eliminate cross-frequency training degradation. An auxiliary conditional generative adversarial network (ACGAN) with kinematic sifting is used to augment and classify human activity data and recognize ASL signs. Future directions for overcoming these challenges and extending the proposed techniques to ASL recognition are discussed.
Mehmet Saygin Seyfioglu, M.S., TOBB University of Economics and Technology, 2017
Mehmet Saygin Seyfioglu
M.S. in Electric-Electronics Engineering, December 2017
Thesis Title: Deep Neural Network Initialization and Training Methodologies for Radar Micro-Doppler Signature Classification
Abstract: Recently, deep neural networks (DNNs) have been the subject of intense research for the classification of radio frequency (RF) signals, such as synthetic aperture radar (SAR) imagery or micro-Doppler based automatic target recognition. However, a fundamental challenge is the typically small amount of data available due to the high costs and resources required for measurements. Deep neural networks (DNNs), however, require large-scale labeled datasets to prevent overfitting while having good generalization. In this thesis, the efficacy of two neural network initialization techniques- unsupervised pre-training and transfer learning- for dealing with training DNNs on small data sets are compared. Unsupervised pre-training is implemented through the design of a convolutional autoencoder (CAE), while transfer learning from two popular CNN architectures (VGGNet, GoogleNet and ResNet). Also, a novel method for generating diversified radar micro-Doppler signatures using Kinect-based motion capture simulations is proposed as a training database for DNNs exploiting transfer learning. Results have also been compared with the conventional approach where handcrafted features are employed to train an SVM classifier. In particular, it is shown that the proposed residual transfer learning network, DivNet, offers the highest classification accuracy among other DNN architectures and, is robust to image noise.
Halil Ibrahim Sumer, M.S., TOBB University of Economics and Technology, 2016
Halil Ibrahim Sumer
M.S. in Electric-Electronics Engineering, January 2016
Thesis Title: Indoor Fall Detection Using a Network of Seismic Sensors
Abstract: Falls present a great health threat as people get older; moreover, studies have shown that rapid response is critical to decreasing fall-related mortality. Thus, the development of signal processing algorithms for sensors used in biomedical applications involving assisted living has become an avid area of research. In this work, a novel algorithm for activity classification and fall detection using a seismic sensor network is proposed. More specifically, classification of falling as well as sources of parasitic signals, such as dropping an object, slamming a door, and shutting a window, are considered. A new target detection and feature extraction algorithm based on wavelet coefficient characterization and spectral statistics is proposed. Results quantifying the performance of the algorithm on real data from a seismic sensor network are given. It is shown that the algorithm offers a reduction of false alarms especially in the case of potentially confusable parasitic signals. According to the studies human falls were detected with an accuracy rate of 99.4%.
Baris Erol, M.S., TOBB University of Economics and Technology, 2015
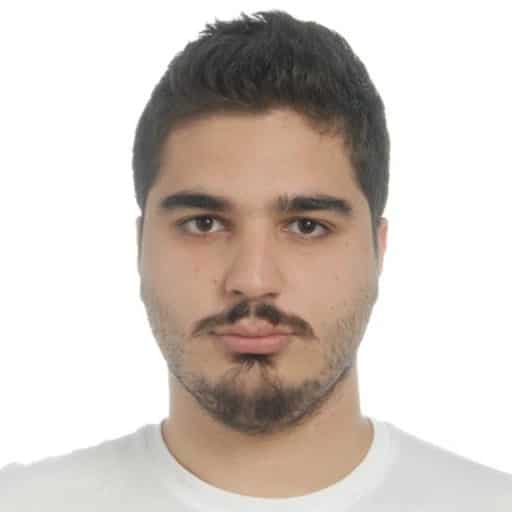
Affiliation:
Senior Research Scientist,
Siemens, Michigan
Earned his PhD in ECE from Villanova University under mentorship of Dr. Moeness Amin
Baris Erol
M.S. in Electric-Electronics Engineering, August 2015
Thesis Title: Adaptive Micro-Doppler Data Processing for Environmental and Operational Conditions
Abstract: A key challenge for radar survelliance systems is the discrimination of ground based targets, especially humans from animals, as well as different types of human activities. For this purpose, target micro-Doppler signatures have been shown to yield high automatic target classification rates; however, performance is typically only given for near-optimal operating conditions using a fixed set of features. Over the past decade a vast number of micro-Doppler features have been proposed for classification of radar micro-Doppler signatures. In fact utilization of all possible features does not guarantee maximum classification performance and the selection of an optimal subset of features is scenario dependent. In this work, a comprehensive survey of micro-Doppler features and their dependence upon system parameters and operational conditions – such as transmit frequency, range and Doppler resolution, antenna target geometry, signal to noise ratio, and dwell time – is given. A new feature design for human micro-Doppler signatures is proposed. Algorithms for optimizing classification performance for a reduced number of features are presented and it is shown that a well selected subset of robust features yields better results. Performance gains achievable using adaptive feature selection are assessed for a case study of interest.
Bahri Cagliyan, M.S., TOBB University of Economics and Technology, 2015
Bahri Cagliyan
M.S. in Electric-Electronics Engineering, August 2015
Thesis Title: Human Micro-Doppler Classification Using a Low Cost, Wireless Radar Mote for Health Monitoring
Abstract: In recent years, radar applications aiming health monitoring have been increasingly investigated. Infrared, acoustic, vibration/seismic, and visual sensors have been used for the recognition of human activities. Unlike these sensors, radars oer unique advantages over ether sensors for recognition and classification of human activities, such as operating virtually all weather conditions, increased range, and better coverage. Human activity monitoring using radars is gaining importance for detection of emergency situations in home and clinical environments. In this thesis, normal human activities such as walking, running , crawling and elderly and disabled human activities such as walking with a cane, walking with a limp, walking with a walker, and wheelchair will be observed with a radar and differences will be discussed. Effects of aspect angle and dwell time on classification success rate will be presented for these activities.
Cesur Karabacak, M.S., TOBB University of Economics and Technology, 2015
Cesur Karabacak
M.S. in Electric-Electronics Engineering, April 2015
Thesis Title: Enhancement of Radar Micro-Doppler Classification Performance in Noisy Conditions
Abstract: Micro-Doppler radar signals for different target types or different movements of a target are also different from each other. This leads to usage of micro-Doppler radar signals as a source of classifying targets. Many micro-Doppler based target classification studies have been published in the last ten years. The studies which are done using experimental radar data are usually carried out in situations where target is close to the radar and clutter effect is minimal. In simulation studies, noise and clutter effects are also ignored. Measurement of classification performance for idealistic conditions in which noise and clutter effects are not taken into account makes the outcomes of the study doubtful. In this thesis, a classification study of micro-Doppler based target signatures is carried out using simulation data. While generating radar simulation data of targets, noise and clutter effects are also modeled. Classification performance obtained from the data containing noise and clutter is compared to noise free data. In addition, a method is proposed to increase the performance in noisy situations using image processing techniques. The results are obtained with two different classifiers and the proposed method has made an increase from 5% to 15% in the classification performance.
Burkan Tekeli, M.S., TOBB University of Economics and Technology, 2015
Burkan Tekeli
M.S. in Electric-Electronics Engineering, January 2015
Thesis Title: Information Theoretic Feature Selection for Human Micro-Doppler Classification
Abstract: The micro-Doppler signatures resulting from vibration or rotation of parts of a moving target can be used to not just classify different targets like vehicles, tanks, helicopters, or even animals, but also to classify and recognize different activities. For this purpose, a plethora of features have been extracted and proposed in the literature for classification of micro-Doppler signatures. Yet, use of all features does not guarantee the optimal classification performance. A high classification performance or success rate can be better obtained using a subset of features, which are selected among all possible features. In this thesis, this situation is demonstrated by comparing results on classification performance obtained with not only a selected subset of features, but also using all features for human activity classification. More specifically, information theory based feature selection algorithms are examined and compared for selecting features having optimal classification performance. Feature selection is considered for changing radar-target geometries aspect angle as well. Results Show that when the aspect angle is known mRMR algorithm yields higher correct classification rates, while for unknown angles MIFS-U algorithm performs better. When feature selection is used %1,1 improvement is achieved over when all features are used. Work provides basis for adaptive selection of features under varying operational conditions.
Sajjad Baghaee, M.S., Middle East Technical University, 2012
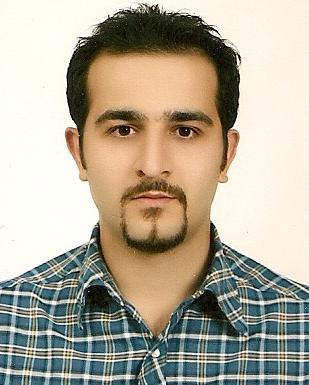
Affiliation:
IoT Engineer,
JEOIT Internet Teknolojileri Bilgisayar Bilisim Muhendislik Enerji Ticaret Limited Sirketi,
Ankara / Turkey
Earned his PhD in ECE from Middle East Technical University under mentorship of Dr. Elif Uysal
Sajjad Baghaee
M.S. in Electric-Electronics Engineering, June 2012
Main Advisor: Elif Uysal [Co-Advisor: Sevgi Z. Gurbuz]
Thesis Title: Identification and Localization on a Wireless Magnetic Sensor Network
Abstract: This study focused on using magnetic sensors for localization and identification of targets with a wireless sensor network (WSN). A wireless sensor network with MICAz motes was set up utilizing a centralized tree-based system. The MTS310, which is equipped with a 2-axis magnetic sensor was used as the sensor board on MICAz motes. The use of magnetic sensors in wireless sensor networks is a topic that has gained limited attention in comparison to that of other sensors. Research has generally focused on the detection of large ferromagnetic targets (e.g., cars and airplanes). Moreover, the changes in the magnetic field intensity measured by the sensor have been used to obtain simple information, such as target direction or whether or not the target has passed a certain point. This work aims at understanding the sensing limitations of magnetic sensors by considering small-scale targets moving within a 30 cm radius. Four heavy iron bars were used as test targets in this study. Target detection, identification and sequential localization were accomplished using the Minimum Euclidean Distance (MED) method. The results show the accuracy of this method for this job. Different forms of sensor sensing region discretization were considered. Target identification was done on the boundaries of sensing regions. Different gateways were selected as entrance point for identification point and the results of them were compared with each other. An online ILS system was implemented and continuous movements of the ferromagnetic objects were monitored. The undesirable factors which affect the measurements were discussed and techniques to reduce or eliminate faulty measurements are presented. A magnetic sensor orientation detector and set/reset strap have been designed and fabricated. Orthogonal Matching Pursuit (OMP) algorithm was proposed for multiple sensors multiple target case in ILS systems as a future work. This study can then be used to design energy-efficient, intelligent magnetic sensor networks.