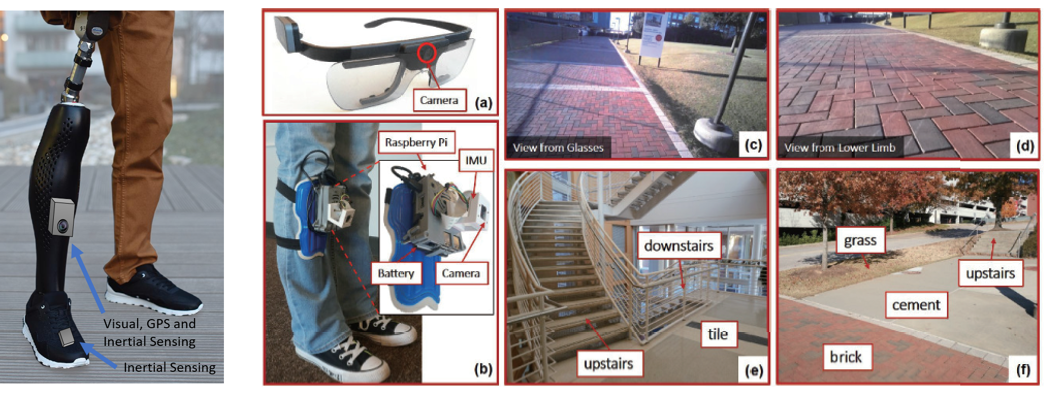
Environmental Context Prediction for Lower Limb Prostheses
Reliable environmental context prediction is critical for wearable robots (e.g. prostheses and exoskeletons) to assist terrain-adaptive locomotion. This paper proposed a novel vision-based context prediction framework for lower limb prostheses to simultaneously predict human’s environmental context for multiple forecast windows. By leveraging Bayesian Neural Networks (BNN), our framework can quantify the uncertainty caused by different factors (e.g. observation noise, and insufficient or biased training) and produce a calibrated predicted probability for online decision making. We compared two wearable camera locations (a pair of glasses and a lower limb device), independently and conjointly. We utilized the calibrated predicted probability for online decision making and fusion. We demonstrated how to interpret deep neural networks with uncertainty measures and how to improve the algorithms based on the uncertainty analysis. The inference time of our framework on a portable embedded system was less than 80ms per frame. The results in this study may lead to novel context recognition strategies in reliable decision making, efficient sensor fusion and improved intelligent system design in various applications.
This work was a collaboration between the ARoS Lab and the Neuromuscular Rehabilitation Engineering Laboratory (NREL) at UNC Chapel Hill / NC State. This work was supported by the National Science Foundation under award 1552828, 1563454 and 1926998.